How to leverage peptide design strategies to increase your candidate’s stability and reactivity
Peptides are uniquely poised between small and large molecules and, therefore, their unique structure and reactivity make them desirable for many applications. A good peptide design strategy starts by determining potential bioactive candidates, followed by sequence optimization to increase the stability and reactivity of these molecules. In this article, we will highlight the most crucial steps in the development of new peptides.
What are the guiding principles of peptide design?
The versatility of peptides has propelled the inclusion of these molecules in multiple applications:
- Proteomics: protein-protein interaction, protein-substrate interaction, biomarker screening, NMR studies, inhibition studies
- Drug delivery: cell-penetrating peptides, self-assembling peptides for drug delivery
- Vaccine development: peptide vaccines
- Antibody development: peptide antibody development – as binders for library screening or as antigens for animal immunization; affinity purification of monoclonal and polyclonal antibodies
- Drug development: therapeutic peptides, antimicrobial peptides
- Tissue engineering: self-assembling peptides for tissue engineering, cell-adhesion peptides, etc.
- Cosmetics: anti-aging, mitigation of skin and hair conditions, etc.
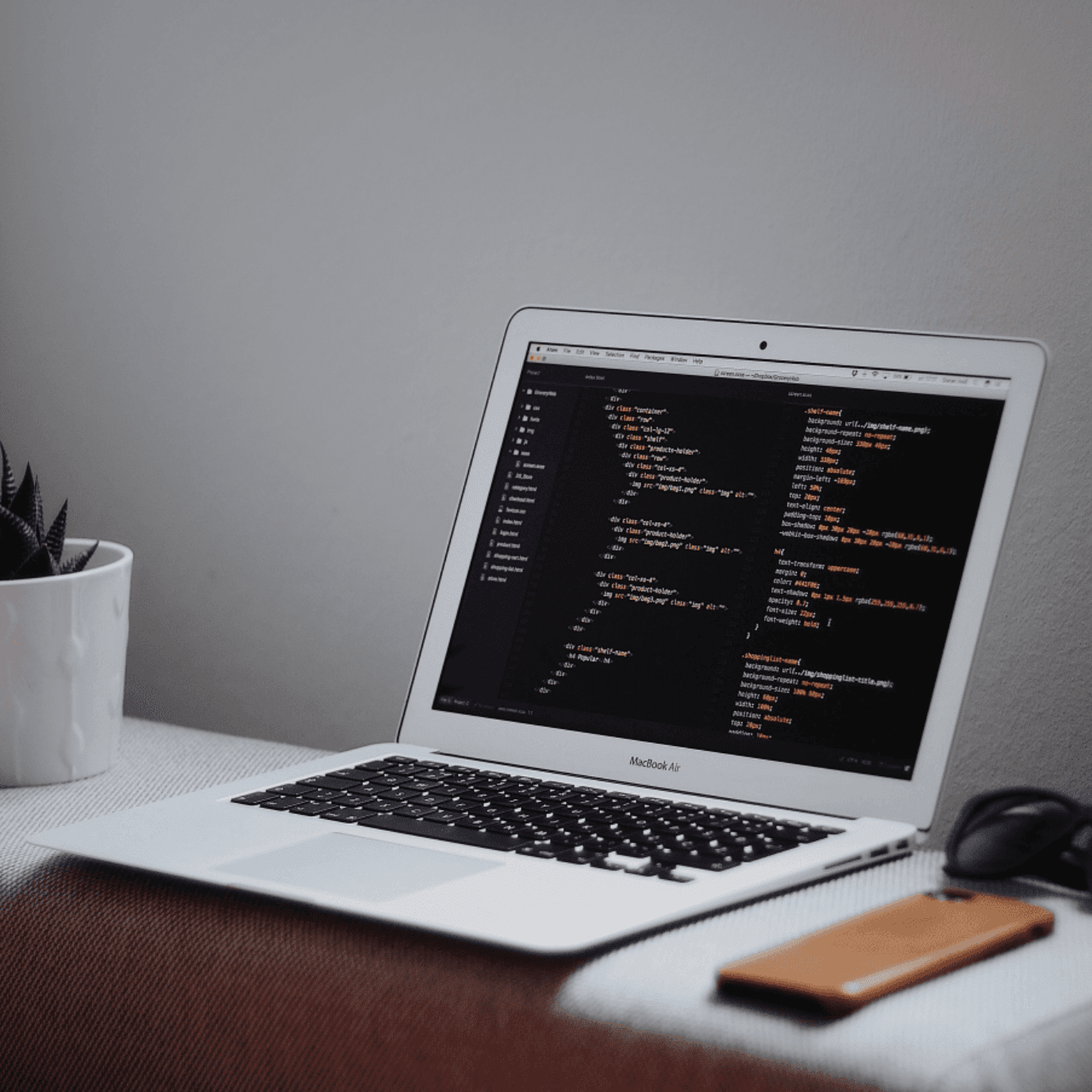
Chemical synthesis is usually the preferred route for peptide synthesis. These methods also allow the careful optimization of peptide’s solubility and stability.
Several principles can be taken into account to optimize the properties of a peptide:
- Length: the purity of a chemically synthesized peptide decreases with sequence length, hence, peptides with 6-20 residues (optimal 15) are ideal, this keeps the production costs low since peptides of this size are easier to produce and more straightforward to purify, for longer peptides, fragment ligation or recombinant production should be considered.
- Solubility: most peptides originated from native proteins are generally hydrophobic, a way to reduce its hydrophobicity is by replacing non-essential hydrophobic residues with charged or polar residues (i.e. aspartic acid, lysine, arginine, histidine, glutamic acid) or by shortening the amino acid sequence.
- Crosslinking: peptides rich in certain amino acids (aspartic acid, glutamic acid, histidine, lysine, asparagine, glutamine, arginine, serine, threonine, tyrosine) are prone to establishing hydrogen bonds, and forms gels in solution; in this way, a high proportion of these residues should be avoided in the final sequence.
- Stability: the amino acid composition of a peptide has great influence over a peptide’s overall stability. The stability of a native peptide can be increased by replacing residues on the peptide backbone, by applying a chemical modification strategy (acetylation, amidation, etc.) or by conjugating peptides with other molecules (e.g. PEG or BSA). Common examples of residues that reduce the overall stability of a peptide include:
- Multiple cysteine and methionine residues may render a peptide that is highly susceptible to oxidation, this can be avoided by replacing the cysteine with serine and the methionine with norleucine
- N-terminal glutamine residues causes the peptide to undergo undesirable cyclization in acidic conditions
- Bonds containing aspartic acid linked to glycine, proline or serine are highly susceptible to hydrolysis, thus, Asp should be replaced in these situations
- Adjacent serine or proline residues increase the chances of deletion, decreasing the purity of the final product
- Secondary structure formation, such as β-sheets, considerably reduce the stability of the peptide
How do we obtain the sequences for peptide design in the first place?
Traditionally, peptides originate from native protein sequences. These sequences can be chemically modified or conjugated with other molecules to increase stability. But they can also be modified by random or site-specific modifications in the attempt to increase the specificity of a peptide towards its intended target.
More recently, peptide research is increasingly evolving to expand the functionality of these conventional peptides by developing de novo peptide design approaches. These approaches, often based on deep learning algorithms, promise to revolutionize synthetic biology by granting new peptides unique reactivities and novel functionalities.
How do we ensure the bioactivity of a peptide?
The stability of a peptide can easily be predicted during the peptide design stage. However, its bioactivity is harder to estimate.
In silico prediction software has become an increasingly popular way to predict the bioactivity of synthetic and natural peptides.
However, in the absence of structural information of the target molecule, bioactivity may be impossible to predict using in silico methods alone.
In this way, it is often desirable to use these methods to guide the determination of potential bioactive proteins for the design of peptide libraries. But instead of using them to find the ideal candidate, they can be used to design a peptide library with the most suitable candidates. These libraries, comprising a systematic combination of a large number of different peptides, are compatible with high throughput screening techniques and, therefore, can accelerate peptide discovery.
Peptide libraries can be synthetically generated or created by phage display. However, the synthetic format offers many advantages including ease of library creation and possibility to include unnatural modifications and residues into the peptide backbone.
Concluding remarks
Peptide design and discovery is increasingly being used to expand the functionalities of natural peptides for multiple applications. These strategies should take into account both peptide stability and bioactivity to obtain the best possible candidates.
However, even the most effective in silico design strategies should include an experimental validation step. For this reason, rational peptide design and library screening should go hand-in-hand to maximize the stability and bioactivity of every new biomolecule.
- Jenson, J. M. et al. Peptide design by optimization on a data-parameterized protein interaction landscape. Proc Natl Acad Sci U S A. 2018; 115(44):E10342-E10351. doi: 10.1073/pnas.1812939115
- Tran, N. H. et al. De novo peptide sequencing by deep learning. Proc Natl Acad Sci U S A. 2017; 114(31):8247-8252. doi: 10.1073/pnas.1705691114
- Tran, N. H. et al. Deep learning enables de novo peptide sequencing from data-independent-acquisition mass spectrometry. Nat Methods. 2019; 16(1):63-66. doi: 10.1038/s41592-018-0260-3
- Trasatti, J. P. et al. Rational design of peptide affinity ligands for the purification of therapeutic enzymes. Biotechnol Prog. 2018; 34(4):987-998. doi: 10.1002/btpr.2637